Nearly a month and a half ago, the sports enthusiast community was consumed with the annual tradition of filling out their NCAA brackets and predicting the outcome of the tournament. I was watching a segment on Sportscenter about filling out your bracket, and they showed an experiment in which a group of people were brought into a room and each asked to fill out a bracket using just the seed numbers...no team names were shown. In other words, these people were randomly picking winners based only on seed. I don't remember the exact results, however the host of the segment was surprised at the accuracy and I seem to remember that the crowd did better than any of the other techniques for predicting the tournament that were shown in that same segment. (Unfortunately, I can't find any video of this segment to link to...)
The segment inspired me to try something similar, but I wanted my "crowd" to be a little more informed than the people participating in the ESPN segment. My idea was to show people a small set of facts about the two teams participating in a game, so that they could make a more informed decision about the winner. I chose not to show the team names as well, so that any bias for or against well-known teams (e.g., Duke) would not be a factor.
I also needed a crowd of people to answer these questions, and I chose to use
Amazon's Mechanical Turk service to provide that crowd. For those of you who may not be familiar with Mechanical Turk, it is a service where people can post typically small simple tasks and have other people perform these tasks. The typical task also requires some sort of human judgement that can't easily be performed by a computer, such providing a label for an image, filling out a CAPTCHA, etc. A monetary value is also assigned to each task, though these values are often quite small (e.g., $0.01) depending on the difficulty of the task.
Here's how I set up my prediction system:
First, I collected 22 facts about each team participating in the tournament. This was actually the most time-consuming aspect of the entire process. The facts I collected were:
- Bracket (Midwest, East, West, South)
- Seed
- Conference
- Overall Record
- Conference Record
- RPI Rank
- Strength of Schedule Rank
- Conference Rank
- Record Against the Top 25
- AP Poll Ranking
- Coaches Poll Ranking
- Average Points/Game By Leading Scorer
- Points Scored Per Game
- Points Allowed Per Game
- Home Record
- Away Record
- Most Recent Streak
- Turnovers Per Game
- Team Field Goal %
- Team Free Throw %
- Team 3 Point %
- Total Points Scored
Second, I created a program to create tasks that would be posted to Mechanical Turk from the facts that I collected. For each game, the program created 10 tasks. Each task presented 10 random facts about the two teams in a game, and then asked the turker (what people who perform tasks on Mechanical Turk are called) to answer three questions:
- A question about the presented facts of the form, "Which team has a better X?" The purpose of this question is to force the turker to read the facts and filter out any responses from turkers that did not read the facts. This question was inspired by Aniket Kittur et al.'s seminal paper on using Mechanical Turk for usability studies at CHI 2007 (pdf).
- A prediction for a winner between the two teams.
- A prediction of the final score between the two teams. This question also turned out to be filter for turkers that were not sufficiently knowledgeable about basketball. For example, responses with nonsensical or highly unlikely scores (usually far too low, such as 15-7) could be excluded.
Here's a screenshot of one of the tasks that I posted on Mechanical Turk.
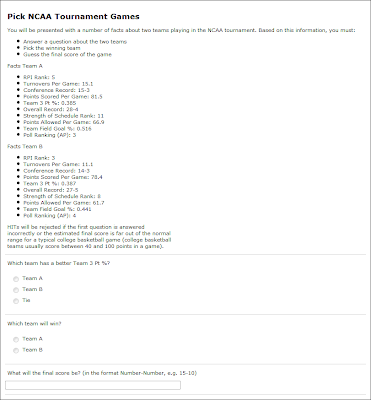
Using this program, I collected answers from turkers starting with the first round of games in the tournament. Winners were determined based on which team the majority of turkers had picked, with each turker response weighted equally. Once I had winners for each game in a round, I generated and ran a new set of tasks for the subsequent round until the entire bracket was filled out.
Tasks were typically posted for 2-6 hours and I paid $0.02 to $0.05 per prediction depending on how quickly I needed predictions submitted (paying more will often lead to quicker responses, but there is evidence suggesting it can also lead to inferior predictions). I ended up paying more for the predictions in the later rounds because I wanted to complete the bracket before the tournament games began (so I could enter the bracket in ESPN's tournament challenge) and I was running out of time to make that deadline.
The final bracket that the turkers selected is shown below, along with the correctness of their final predictions.
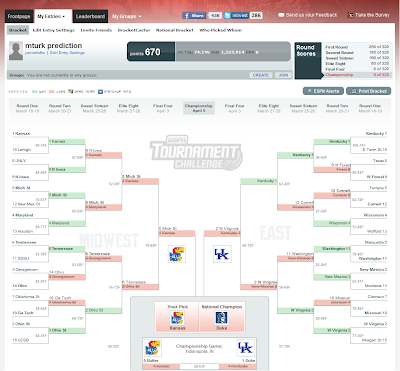
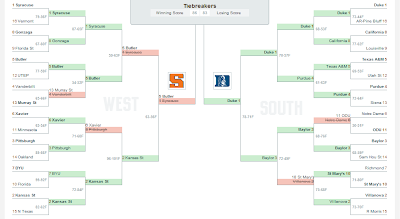
You may also still be able to
view the bracket on ESPN's web site.
The first thing to notice is that the turkers primarily picked winners based on seed, which I found a little disappointing.
Turkers did pick a few upsets however. The first round upsets are particularly interesting, where the turkers picked the following teams against seed:
- 9th seed Northern Iowa over 8th seed UCLA
- 11th seed Washington over 6th seed Marquette
- 10th seed St. Mary's over 7th seed Richmond
Interestingly, all of these upsets actually happened. The upsets are also not just from the 8/9 games in which the two teams are generally evenly matched, but from games with larger differences in seeding. Of course, the turkers missed a number of other upsets that happened in the first round (they missed 7 picks overall).
In the second round, turkers picked only 1 upset, 5th seed Butler over 4th seed Vanderbilt. Butler did win the game, though they were playing a 13th seed Murray State team that had eliminated Vanderbilt in the first round. Again, while accurately picking one upset that did happen, the turkers also missed many other upsets, including Northern Iowa's surprising upset of Kansas. The turkers missed 7 picks overall in the second round, though interestingly only one of these was a carry-over from a missed pick in the first round (Georgetown). In other words, all but one of the teams that the turkers missed in the first round went on to lose in the second round.
In the third round, the turkers finally picked an upset that did not happen, though they also correctly picked one upset. The upset that actually occurred was 3rd seed Baylor over 2nd seed Villanova, though of course in the actual tournament Villanova was eliminated by 10th seed St. Mary's in the second round and Baylor beat St. Mary's in the third round. The upset that turkers picked but did not happen was 3rd seed New Mexico over 2nd seed West Virginia. West Virginia won that game, although they beat the 11th seed Washington, which had eliminated New Mexico in the previous round. As in the previous rounds, the turkers also missed several upsets. Turkers missed 4 picks overall in the third round, with two being carry-overs from misses in the 2nd round (Kansas and the missed upset New Mexico).
In the remaining rounds, the turkers went by seed and selected all #1 seeds to make it into the final four. Only Duke actually made the final four, and that was final correct pick that the turkers made. The turkers had Kentucky and Kansas in the championship game with Kansas winning it all, and obviously that did not happen in the real tournament.
While I was disappointed that the turkers picked primarily by seed, there are two features of their predictions that are interesting. First, it is impressive to me that nearly every upset winner that they chose actually won. This suggests that maybe this Mechanical Turk approach has some value for picking likely upsets based on factual data. Second, the turker picks suffered only five "carry-over misses" across their entire bracket, and three of those were due to the early exit of Kansas. This means that even when turkers were wrong about a team, that team would then likely lose in the following round. This may simply be an artifact of picking primarily by seed, but it is an advantageous feature in any bracket.
There are a bunch of interesting aspects of the turker data to look at that I haven't spent time on yet. In particular, there are a number of cases where the same turker provided multiple predictions for the same game (there is no way to prevent this using the current Mechanical Turk qualification system). None of the games I looked at were dominated by predictions from a single turker, so it does not seem that this bias significantly affected my results. Each task also used a different randomly chosen set of facts, so it is possible that a turker would not have even realized that they were providing a prediction for a game they had already predicted.
Overall, I paid about $35 in fees to turkers to collect these predictions. Clearly this was not a good investment overall, as the bracket did not win me any money. It was a fun experiment though, and it refreshed my knowledge of Mechanical Turk which I may apply in some additional experiments over the summer.
I'll definitely try this experiment again next year, perhaps with some slight modifications, to see if I get similar, and possibly better, results.
-jn
For empire avenue:
EAVB_JJDZUKIRWC